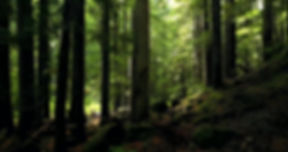
Community Guidelines & Documents
ERGA Governance Document
The ERGA Governance Document builds on the initial consortium structure guidelines and defines ERGA bodies, procedures, roles, and responsibilities. On registration, ERGA Members agree to be bound by both the ERGA Code of Conduct and this Governance Document.
Code of Conduct
ERGA is a bottom-up, open and inclusive community. ERGA membership is open to any individual who supports ERGA’s objectives, registers as a member, and agrees to be bound by the ERGA Code of Conduct.
Privacy Policy
As a community connecting researchers working in the field of biodiversity genomics, ERGA is committed to protecting privacy and respecting privacy regulations, as outlined in the ERGA Privacy Policy.
This document outlines the Open Data Policy (ODP) for ERGA. This includes all data and metadata produced as part of the activities within and/or associated with ERGA.
Sampling Resources
ERGA Sample Manifest
ERGA aims to promote and facilitate ethical and legal sampling practices that are also complete and comprehensive so as to fully document the provenance of all samples. The ERGA Sample Manifest serves as the community standard for documenting sampling.
Community Interest Species Survey
From late 2021 to early 2023 ERGA conducted a community-wide survey to identify species of interest and potential sample providers; the archived list contains ~1700 species of interest.
Data & Tools
The Genomes on a Tree (GoaT) platform is mantained by the Wellcome Sanger Institute. GoaT helps coordinate efforts across the Earth Biogenome Project (EBP) Network at all stages from planning through sequencing and assembly to publication.
Structural Annotation Guide
A step-by-step guide on how to annotate protein-coding genes in your genome. Developed by the Annotation Committee.
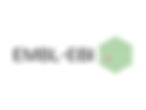
Media
For more information regarding the ERGA brand or other media inquiries please contact us at media@erga-biodiversity.eu.